Unveiling the Carbon Footprint of Machine Learning: Navigating the Environmental Impact
In this post, we're shining a spotlight on the intriguing intersection of machine learning and its environmental implications. We explore the evolution of machine learning, the surge in data storage, the energy demands of colossal models, and the conscientious efforts made by developers to mitigate the environmental footprint of this cutting-edge technology.
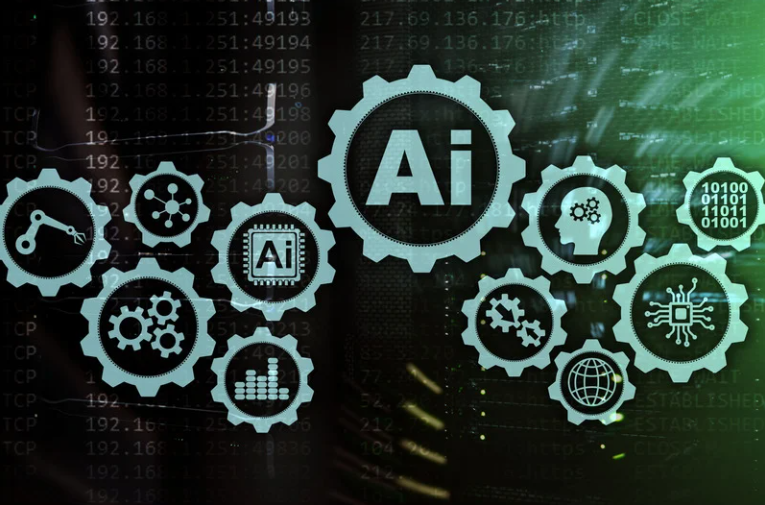
The Evolution of Machine Learning
Over the years, the landscape of machine learning has transformed from an academic pursuit to a practical superpower. The growth in tracked and stored data, coupled with increased computational capacity, has paved the way for groundbreaking models.
A noteworthy example is OpenAI's GPT-3, boasting an impressive 175 billion parameters, a substantial leap from its predecessor, GPT-2, with 1.5 billion parameters. Google Brain's model takes the lead with a staggering 1.6 trillion parameters, highlighting the monumental strides in machine learning capabilities.
Energy Consumption in Machine Learning
However, the advancement in machine learning comes at a cost – a considerable energy demand. The training of these massive models, involving iterative processes and AutoML optimization, contributes to significant energy consumption. Research from 2019 estimated that training a single deep learning model could generate up to 626,000 pounds of CO2 emissions.
To put this in perspective, it is roughly equivalent to the total lifetime carbon footprint of five cars. This estimate is based on energy-intensive models, underscoring the environmental impact of cutting-edge machine learning.
Factors Contributing to Energy Consumption
The surge in energy consumption stems from various factors. Firstly, the expansion of training data sets, with GPT-3's pool reaching around 500 billion words, intensifies the computational demands. Hyperparameter tuning, involving trial and error, adds another layer of energy-intensive processes. Moreover, the production and inference phases, where the models are deployed for practical use, contribute significantly to overall energy consumption. According to Nvidia, 80 to 90% of the cost of a neural network lies in its inference phase.
Watch our Time for Time Series session on Green AI
Green Approaches in Machine Learning
Efforts to address the environmental impact of machine learning commence during the training phase. Traditional methods often rely on design specifics to combat overfitting, but Tangent, a transformative approach, focuses on feature reduction.
By eliminating intercorrelation among features after feature expansion, Tangent enhances model stability, resulting in a more lightweight model with reduced inference computation.
Challenges in Time Series Data
While more data often translates to better results in machine learning, time series data poses unique challenges. The evolving patterns and structural changes in time series data can impair rather than improve model performance. The very nature of time series analysis limits the amount of training data for optimal results, even as storage and processing capacities increase.
Conclusion
This insightful exploration into the carbon footprint of machine learning underscores the delicate balance between technological innovation and environmental responsibility. As Tangent Works leads the way in advancing the field, we remain vigilant in adopting green approaches, ensuring our developments in machine learning, especially in time series data, do not come at the expense of our planet. Our commitment is evident in our Tangent Information Modeler, called Tangent, where we focus on feature reduction and model stability to create efficient, yet environmentally friendly solutions.
As the field of AI continues to evolve, our mission goes beyond mere technological innovation; it involves building an AI ecosystem that is both groundbreaking and sustainable. This balance of advancing AI while protecting our environment is not just our goal - it's our responsibility. At Tangent Works, we are dedicated to leading this charge, proving that the journey towards technologically advanced AI can also be ecologically sustainable.
Article by Henk De Metsenaere, CEO at Tangent Works
Connect with Henk on LinkedIn!
Related posts
Discover further insights: browse related articles.
.png)
Unlock the Power of your Business Data with Tangent
See firsthand how Tangent revolutionizes your approach to data, turning it into actionable predictions that drive success.
Want to add Predictive Power to your software? Get in touch for OEM Solutions