15X
faster time to market
20X
less computing power needed
3x
increase in data science productivity
Meet Tangent, the AI copilot for your daily predictions - delivering instant insights at a fraction of the cost.
Actionable predictions achieved in no time
10-20x faster time-to-market
Finally leverage your abundance of data
Less dependencies, faster results
Time series are different, so are we
The AI copilot designed for your time-series data:
Automated feature engineering
Real-time model (re)building
Seamless integration on your cloud platforms
Rapid deployment
Flexible data pipeline integration
Infinite scaling
100M+
features automatically engineered every month
4M+
predictive models created every month
Your data changes, what about your AI models?
As a data scientist you need to deliver while your data structures evolve. Use the Tangent copilot to efficiently build and operationalize time series models whilst keeping a clear overview.
Increase your productivity
Tangent elevates productivity by swiftly creating powerful data models for forecasting and anomaly detection, acting as a time series AI copilot for data scientists.

Beat time-consuming tasks
Tangent automatically adapts to frequent data changes, a task that normally requires time-consuming, repetitive model creation or tuning. With Tangent, this process is instant, eliminating this traditional bottleneck in data science.

Streamline feature engineering and model operations
Enable effective utilization of predictive analytics with minimal effort and reliable accuracy. Reduce the burden of constantly adapting to data variations and increase your capacity to focus on more strategic tasks.
Tangent is an indispensable tool in an evolving environment, perfect for those who value efficiency, precision, and the intelligent application of AI in data modeling.

Your future is in your data, let’s grab it!
With Tangent's instant predictive model generation, you enable the broad application of predictive analytics without the typical dependencies.
Create highly accurate models in an instant
This easy-to-use platform instantly creates highly accurate models, making advanced AI accessible across various departments. By ensuring precise and timely predictions and forecasts, Tangent significantly enhances operational efficiency and cost-effectiveness.
Catering to the growing need for data-driven decision making
Tangent's streamlined approach and unparalleled explainability of the predictive insights caters to the growing need for data-driven decision-making, positioning Tangent as the go-to AI tool.
Hyper-Granular Predictions at Scale, plugged in your cloud data pipelines
Tangent excels in computing efficiency and flexible deployment for Azure and AWS Cloud environments.
Optimized AI pipelines
Tangent's architecture, optimized for handling large-scale data modelling using minimal cloud resources. Our AI engine facilitates quick integration with existing data platforms and applications.
Easy deployment
Supporting cloud and hybrid setups, Tangent simplifies the incorporation of AI in your data pipelines, ensuring rapid deployment and easy monitoring.
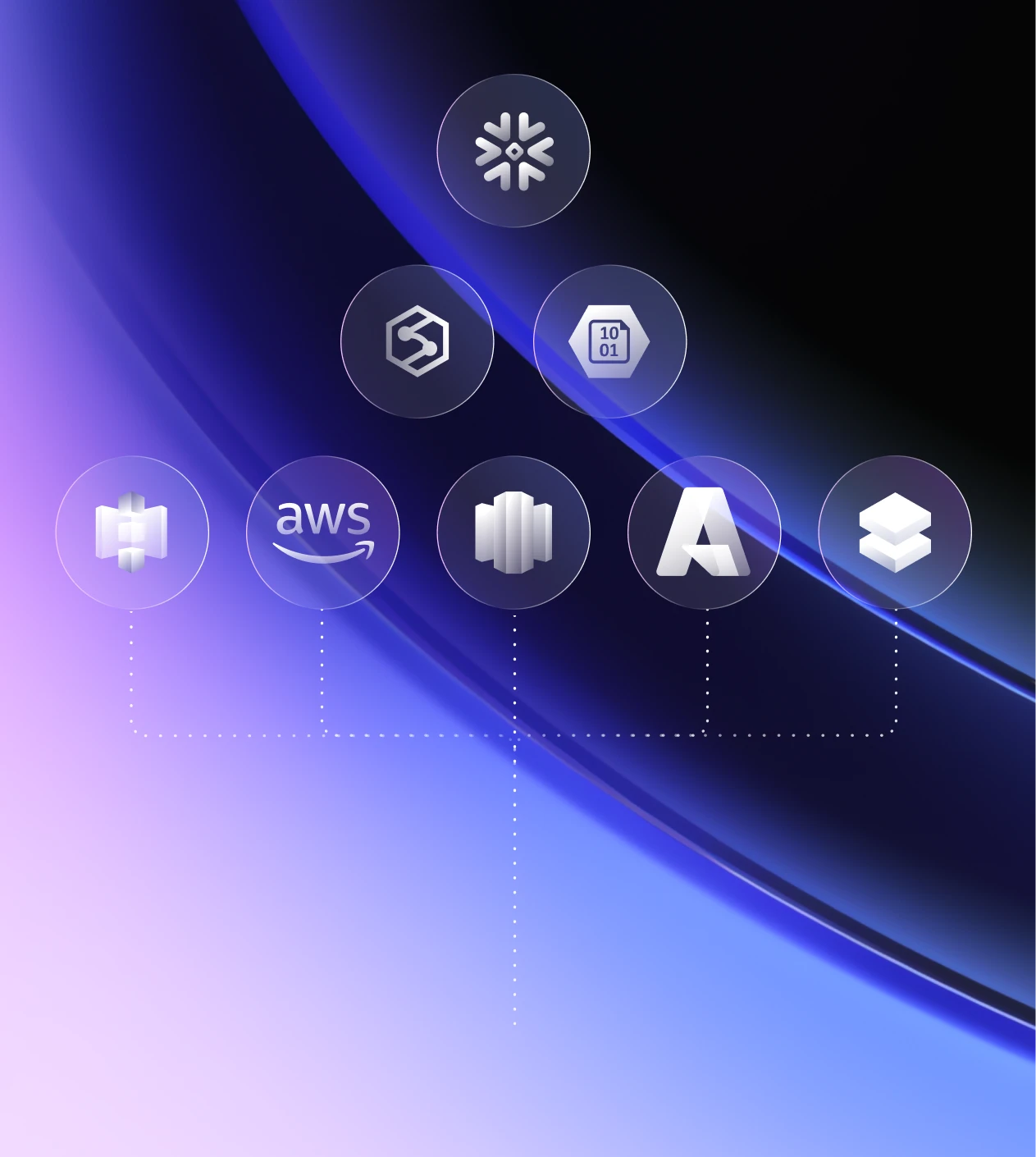
.png)
Unlock the Power of your Business Data with the Predictive AI from Tangent
See firsthand how Tangent revolutionizes time-series predictive analytics, turning your data into actionable predictions at scale.
Want to add Predictive Power to your software application? Get in touch for OEM Solutions
Got questions?
We have answers.
Tangent stands out as the only AI that instantly builds robust predictive models on time-series data, in just minutes. It emphasizes smart, automated feature engineering and selection, achieving efficiency, adaptability, and scalability in AI forecasting. Tangent's models are automatically built, adjusted, and managed, offering excellent baseline performance, enhanced harmonization, maintainability, and business agility.
Tangent excels in various sectors for diverse forecasting needs, from sales and demand to production and price forecasting. It's an all-in-one AI copilot, streamlining forecasts across departments like supply chain, sales, trading, utilities, and production. Utilize Tangent to gain new insights into customers, markets, and operations, harmonizing and scaling all forecasts with one agile solution.
For optimal use of Tangent's hyper-automated AI, data should be clean, organized, and structured. While Tangent can handle some gaps and outliers, better data quality increases the likelihood of instantly deriving predictive value and building effective forecasts. Providing a substantial historical dataset of several months, along with multiple raw features, enhances Tangent's ability to identify complex patterns and correlations. For detailed recommendations on data input for Tangent, please refer to our Documentation.
Tangent is built for easy integration with your existing cloud platforms and data pipelines. It features flexible APIs and connectors for efficient deployment with major data platforms like Azure, Databricks, Snowflake, and AWS. Designed as a versatile building block in your AI landscape, Tangent streamlines and automates time series predictive analytics, from forecasting to anomaly detection.
Implementing and integrating Tangent is straightforward and flexible. New customers receive expert support and templates from Tangent Works for a smooth start. Operationalizing Tangent typically takes days, not weeks or months, unlike many other more complex and compute-intensive solutions.