Resources
Resources
Welcome to our growing collection of resources, featuring a selection of blog posts, webinars, and downloads.
Stay tuned as we continually expand our library!
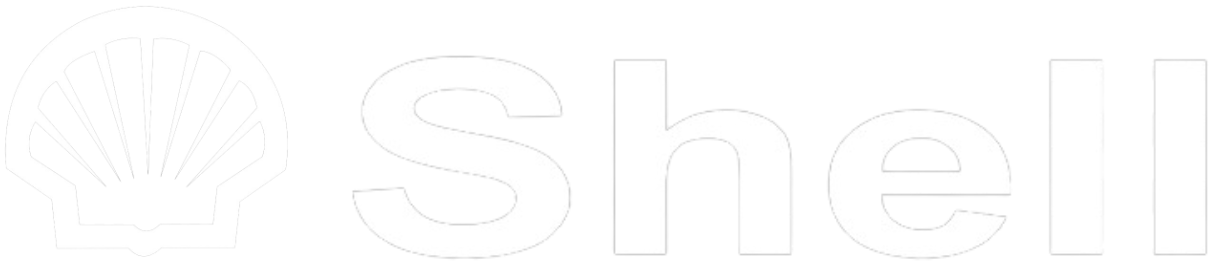
Archive
.png)
Unlock the Power of your Business Data with Tangent
See firsthand how Tangent revolutionizes your approach to data, turning it into actionable predictions that drive success.
Want to add Predictive Power to your software? Get in touch for OEM Solutions